Before the launch of ChatGPT on Nov 30th 2022, people asked about the security implications of language models producing human fluent responses. The range of concerns were difficult to comprehend until the Public had access. The first malicious prompts were reported a few hours after public access, unleashing a wave of public attention on the fairness and safety expected from LLMs.
The fairness and safety guarantees of LLMs, while being crucial to the social impact of its adoption, are equally as important to the cybersecurity challenges they present. Anyone interested in securing or securing adoption of this technology will need to grasp the interplay and distinctions between the concepts of LLM security and LLM fairness.
Security vs. Fairness
Humans and models are collections of actions, behaviors and responses. To say a person is “good” or “bad” is too shallow a classification; the same is true for labeling a model as “biased,” “unfair” or “secure.” It is difficult to articulate what quantifies the threshold of secure and insecure. Nonetheless, there are relative comparisons and imperfect measures, which can guide decision making.
Fairness and security are colloquially interchanged sometimes; however, they are not the same trait. Ensuring fairness when using LLMs is to prevent social harms, particularly to marginalized communities. Security, on the other hand, prevents the LLM from being manipulated to aid malicious intentions.
Ensuring fairness in the model, to prevent social harms, is advancing through numerous collaborative projects across industry, research and non-profit organizations:
- A survey on bias and fairness in machine learning
- The UCLA-NLP lab biography of “awesome fairness papers”
- Exploring how machine learning practitioners (try to) use fairness toolkits.
Our aim at Palo Alto Networks is to provide a companion perspective on security. The term to “secure” a model is still being defined, simultaneously with the discovery of new LLM abilities. Community consensus does not yet exist and governing boards are only just being established.
To illustrate the distinctions and overlap between the fairness and security of an LLM, we describe four scenarios.
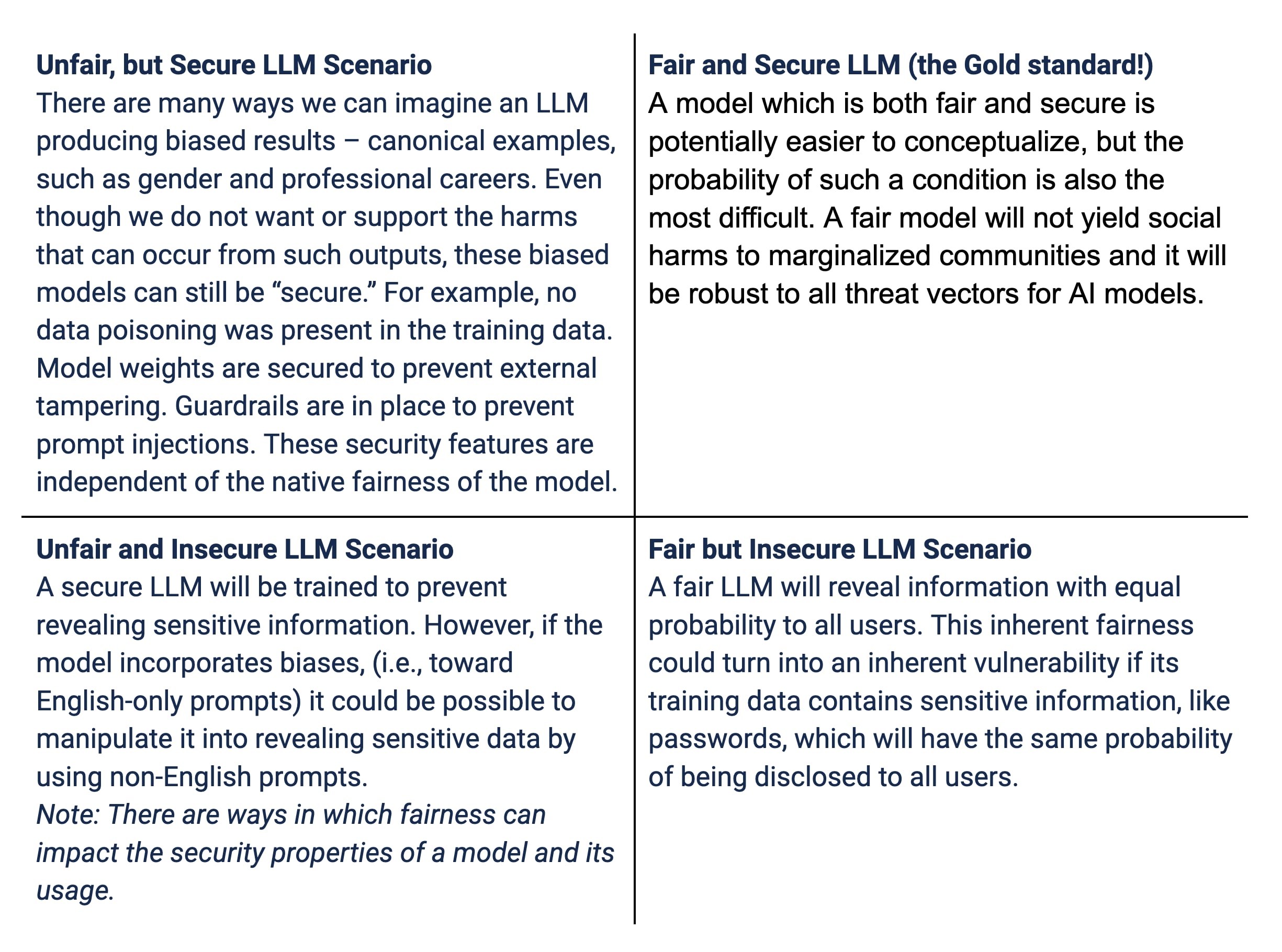
As we evaluate announcements, products, claims etc, the methods to measure, rank and mitigate fairness can relate to a model’s security, but it will not be synonymous.
The best set of measures and processes to evaluate what it means to “Secure AI” remains an open question. As cybersecurity professionals, we recognize that security comes from the system, not the individual components.
LLMs are just part of an ecosystem. Securing AI systems will need to occur both at the component and system level to ensure comprehensive security. For more insights that will empower you to safeguard your systems effectively, read Securing Generative AI: A Comprehensive Framework.